AI is changing learning and development. It's about making training fit each employee perfectly.
Picture this: training that adapts to you.
How does AI do this in L&D? Through smart algorithms and personalized content. Every training session becomes spot-on effective.
And there's more. We'll dive into the leading AI tools in L&D. Plus, how to handle their challenges.
Curious about these game-changers? Let's dive in.
Key Takeaways:
- Customized Learning: AI tailors training to each learner's unique style and needs.
- Immediate Feedback: AI provides real-time guidance, shaping adaptive learning paths.
- Navigating Challenges: Despite challenges in data privacy and adaptation, AI promises exciting developments in immersive and predictive L&D technologies.
Harnessing AI for Enhanced Learning Experiences
AI is reshaping learning and development. It's turning the old-school, one-size-fits-all approach on its head. Now, it's all about personalized learning journeys.
Why? Three reasons:
- Personalized Content: AI knows what you need. It delivers content that fits your style and goals. Imagine a platform that gets you better than you get yourself.
- Real-Time Feedback: Forget waiting for feedback. AI gives it now. This means instant adjustments and better learning strategies.
- Adaptive Learning Paths: Everyone learns differently. AI gets that. It creates unique learning paths for each person.
Personalized Content Delivery
Think of a platform that aligns with your learning style and career goals. AI makes this possible. It uses your data to deliver content that resonates with you.
This isn't just a dream. It's happening in schools and workplaces. AI is suggesting content that matches specific learning goals.
Real-Time Feedback and Guidance
Traditional learning often means delayed feedback. AI changes that. It offers real-time feedback and guidance. This helps learners see where they're at and how to improve.
AI tools are now virtual tutors, offering instant feedback on assignments. This closes knowledge gaps and boosts learner outcomes.
Adaptive Learning Paths
Every learner is unique. AI embraces this. It creates learning paths tailored to individual needs.
This means:
- No overload with irrelevant content.
- Personalized learning experiences.
- Non-linear course progression.
- Optimal learning results.
AI customizes the educational experience. It adapts to individual learning styles and paces. This is how AI personalizes the learning journey for everyone.
Let's look at the AI tools leading this transformation in L&D
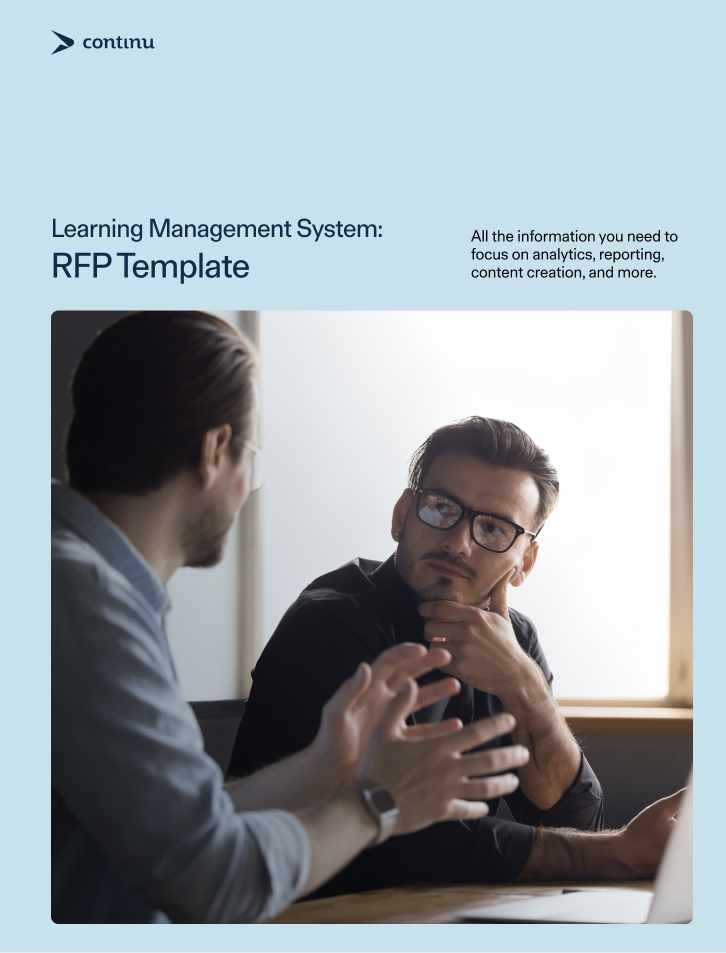
LMS RFP Template - Make The Right Choice
Shopping for an LMS can lead to indecisiveness, wrong choices, and relying on incomplete information.
Download Free CopyAI Tools Transforming L&D Landscape
AI isn't just enhancing learning. It's reshaping how we design and deliver L&D programs. Think virtual assistants, NLP, ML algorithms. They're leading the charge in e-learning.
Here's a closer look at some AI tools for learning and development:
Virtual Assistants and Chatbots
Picture a tutor available anytime. That's what AI-powered assistants and chatbots do. They're like a learner's 24/7 buddy, offering help and motivation. They adapt by analyzing learner interactions.
Siri, Alexa, and Genie from Deakin University are prime examples.
Natural Language Processing (NLP)
NLP lets computers understand human language. It's revolutionizing digital learning. NLP means more natural interactions, personalized feedback, and content creation like essays.
Tools like TextOptimizer and Google Cloud Natural Language API are key players here.
Machine Learning Algorithms
The real power behind AI in L&D. ML algorithms analyze data to spot trends. They help instructional designers make smarter decisions.
These algorithms personalize learning paths based on performance and preferences.
Implementing AI in L&D isn't without its hurdles.
Let's explore how to navigate these challenges.
Overcoming Challenges in Implementing AI in L&D
AI in L&D is a game-changer, but it's not without its challenges. Data privacy, staff training, and balancing human-AI interaction are big hurdles. But don't worry, they're not unbeatable.
Here's how to tackle them:
- Data Privacy and Security: This is huge. AI means lots of data, and keeping it safe is key. Think strong encryption and strict adherence to data laws. Training staff on data privacy is also crucial.
- Staff Training and Adaptation: To make the most of AI, L&D pros need to get savvy with it. They need to know not just the tech but how to use AI to boost learning. And let's not forget easing learners into AI-based methods. It's all about showing the benefits and offering support.
- Balancing Human and AI Interaction: It's about finding the sweet spot. AI brings the smarts, but humans bring the heart. The goal? Blend AI's efficiency with human empathy for killer learning experiences.
Future Trends: AI's Impact on L&D
AI's role in L&D is only going to grow. Get ready for immersive tech, predictive analytics, and collaborative learning environments, all supercharged by AI.
Here's what's coming:
Immersive Technologies
VR and AR are set to make learning super engaging. Imagine learning in virtual worlds or with augmented content. AI will tailor these experiences to each learner.
Predictive Analytics
This is about foreseeing skill needs. AI will help L&D pros create targeted training to fill skill gaps. It's about personalizing training to fit learner needs and performance.
Collaborative Learning Environments
AI will also help build spaces where learners can share knowledge and work together. Think interactive platforms and group projects, all fine-tuned by AI.
Ready to wrap this up? Let's summarize what AI means for the future of learning and development.
Summary
So, we've seen how AI is a game-changer in learning and development. It's not just about making learning better. It's about a total L&D makeover.
Here's the lowdown:
- AI means learning that's tailored, engaging, and on-the-spot helpful.
- But, it's not all smooth sailing. Data privacy, training staff on AI, and finding the right mix of human and AI interaction are challenges we need to tackle.
- Looking ahead, AI's going to keep shaking things up. Think VR, AR, and predictive analytics making learning even more immersive and personalized.
The Bottom Line
AI and L&D are a match made in heaven. Embracing AI is the key to L&D programs that really hit the mark. The future of learning? It's AI all the way.
And that's a wrap! AI in L&D is an exciting journey, and we're just getting started. Ready to dive into this AI-powered future?
Frequently Asked Questions
How is AI used in learning and development?
AI is utilized in learning and development for content creation, personalized learning, adaptive learning, and automating learning management system processes and features. It offers a wide range of applications to enhance the learning experience.
How will AI impact learning and development?
AI will personalize learning materials to suit individual needs, making learning more effective and engaging. Generative AI will also provide real-time feedback and assessments to identify areas for additional support.
What are some AI tools that are transforming the L&D landscape?
AI tools like virtual assistants, natural language processing, and machine learning algorithms are transforming the L&D landscape by enhancing content delivery, automating content creation, and analyzing data to optimize training programs. These tools are revolutionizing how learning and development are approached.
What are the challenges in implementing AI in L&D?
Implementing AI in L&D poses challenges like data privacy, training for L&D professionals, and finding a balance between human expertise and AI capabilities. These are crucial aspects to consider in order to successfully integrate AI into L&D.
How can organizations overcome the challenges in implementing AI in L&D?
To overcome the challenges in implementing AI in L&D, organizations should focus on robust data protection, provide training for L&D professionals, and balance human expertise with AI capabilities. This will ensure smoother integration and effective utilization of AI technologies.